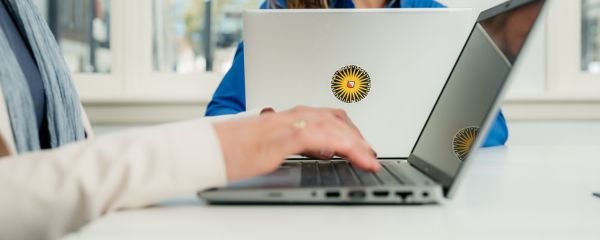
Statistics provides grip on data and models
Before unleashing an algorithm on data, you want to be sure that the data are appropriate for the query as well as that the right query is being asked. That is the challenge Laurence Frank focuses on from Methods & Statistics. She coordinates education for professionals in data science & AI. Together with Rebecca Kuiper, who coordinates the programming courses in the Utrecht Summer School, she shares her insights on the role of statistics in the development of data science models and the challenges involved.
Artificial intelligence is all about data and sophisticated, often self-learning algorithms. ‘An advanced algorithm can recognise complex patterns, but without the right statistical methods you risk getting stuck in the exploratory phase and drawing wrong conclusions,’ Laurence Frank explains. “Statistics helps determine whether the data is representative of the target audience, whether data is missing and whether bias plays a role. It provides an awareness process that is essential to get valid answers.”
Getting a grip with CRISP-DM
“People often think that more data is better, but that is a misconception. Even with big data, certain groups can be missing. Older people are often less represented online, for example. You have to be aware of that and do checks on that,” Laurence Frank says. To avoid these pitfalls, in the courses she uses the CRISP-DM method, a step-by-step approach for data science projects. “That method is not perfect, but it does incite good thinking. If you use this method, you can at least be sure that all the important questions have been addressed.”
Rebecca Kuiper adds: “It helps you work in a structured way and perform the right checks at the right time. It prevents you from just looking for connections without having a clear research question. So you avoid accidental discoveries that don’t hold up when repeated.”
Cyclical processes
Data science and AI development are cyclical processes. Each analysis raises new questions. You have to go through the cycle again and again: collect data, check it (including ethical checks), sharpen your research question. This ensures that you get a grip and know what you are doing. Have you done an analysis and get results you didn’t expect? Then that raises new questions. You enter the cycle again: checks again, another refined research question or an update thereof. By following the systematics, you can take a business-like approach and plan. You get signals in time if things are not going well.
Programmes
Data science and AI development are cyclical processes. Each analysis raises new questions. You have to go through the cycle again and again: collect data, check it (including ethical checks), sharpen your research question. This ensures that you get a grip and know what you are doing. Have you done an analysis and get results you didn’t expect? Then that raises new questions. You enter the cycle again: checks again, another refined research question or an update thereof. By following the systematics, you can take a business-like approach and plan. You get signals in time if things are not going well.
'Compare it with vaccines'
Where will the use of statistical methods for AI be in five years? Laurence Frank does not expect the need to change substantially. “We will still find it important then that data is of good quality, that there is no bias in the models and that we make valid statements. I expect the importance of this will only increase. I hope we will start working more and more systematically. Compare it with vaccines, for example. There, the steps to approval are carefully defined and everything is recorded. For AI, such an approach is lacking in practice, while the consequences can be huge if things go wrong. I hope that, as with vaccines, we will eventually develop a methodology whereby AI is only released on humans after careful development and testing phases. This realisation is strong in the Netherlands and Europe, but this AI development has gone so fast.”
More information?
Read about responsible AI practices and AI Labs. Or follow one of Utrecht University’s courses and training programmes
Methods and Statistics is part of the Department of Social Sciences in the Faculty of Social Sciences. These scientists have the knowledge to use data to answer research questions that advance science and society.
The Utrecht Summer School is a collaboration between Utrecht University, Utrecht University of Applied Sciences, HKU and the University for Humanistics. The Utrecht Summer School offers some 160 courses in summer as well as in winter, aimed at students and professionals. Twenty of these are on methods & statistics.